Statistical Learning in Computational Biology
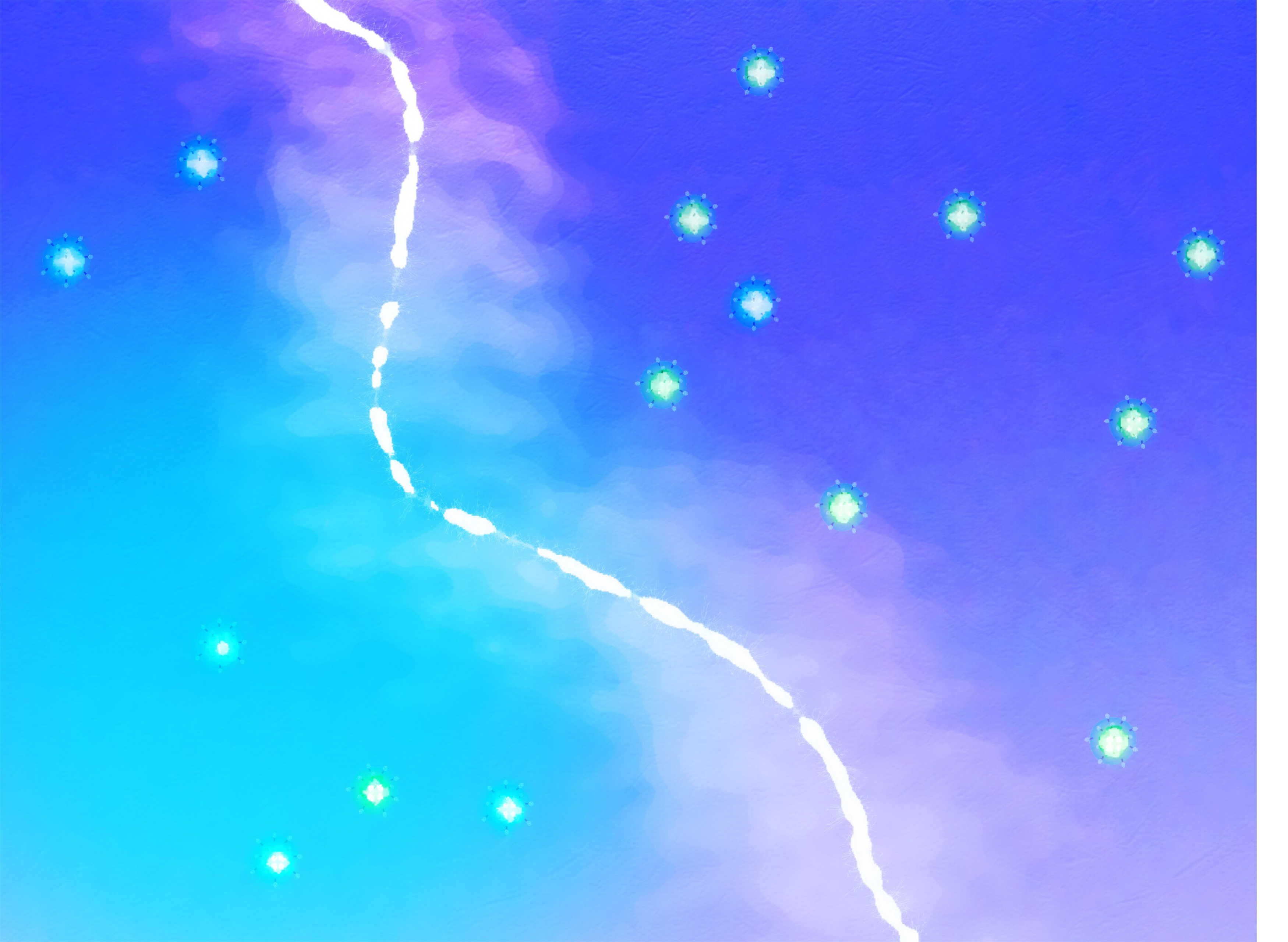
Recent advances in high-throughput technologies have led to an exponential increase in biological data (such as genomic, epigenomic and proteomic data). To find meaningful insights in such large data collections, efficient statistical learning methods are needed. In January 2013 the group "Statistical Learning in Computational Biology" was established at the Department of Computational Biology and Applied Algorithmics. The group is headed by Dr. Nico Pfeifer. We are interested in developing and applying new machine learning / statistical learning methods to solving computational biology problems and answering new biological questions. Previously, we focused on proteomic data, but now the focus is more on epigenomic and genomic data.
Application areas include the study of viruses like HIV, Hepatitis C or Influenza as well as the field of epigenetics. Method-wise we are interested in
- integration of heterogeneous data sets
- improving interpretability of non-linear estimators
- efficient learning methods for large data sets
All those topics can be subsumed under the category "Machine learning for precision medicine".
News
- 6th of May 2017: Nico gave a talk at the Arevir meeting in Cologne.
- 18th of April 2017: Our paper on predicting long-range chromatin interactions (first author Sarvesh, last author Nico) just got published.
- 7th of March 2017: Nico gave a keynote talk at the 1st European Virus Bioinformatics Center meeting (http://evbc.uni-jena.de/meetings/1st-evbc-meeting/).
- 16th of January: Our latest analysis of the 10-1074 trial was just published at Nature Medicine: Antibody 10-1074 suppresses viremia in HIV-1-infected individuals.
- 1st of January 2017: Nico is now full professor for 'Methods in Medical Informatics' at the Department of Computer Science of the University of Tübingen.
- 20th of December: Our work (first author Matthias, last author Nico) on HIV-2 coreceptor usage prediction has just been published at Retrovirology: go to journal homepage
- 13th of December: Our latest analysis of the 10-1074 trial was just accepted at Nature Medicine.
- 9th of December: Nora gave a spotlight presentation at the NIPS workshop on Machine Learning for Health.
- 22nd of June: Our Nature paper was just published: HIV-1 antibody 3BNC117 suppresses viral rebound in humans during treatment interruption
- 16th of June: Nico presented the 2016 research project funded by the supporting members of the Max Planck Society during the annual meeting of the Max Planck Society.
- 15th of June: Our manuscript submitted to Nature just got accepted. More details after the press embargo.
- 16th of May: Our Nature Medicine paper was just published: Impact of Pre-adapted HIV Transmission
- 5th of May 2016: Our Science paper was just published: HIV-1 therapy with monoclonal antibody 3BNC117 elicits host immune responses against HIV-1
- The new semester started. Check out our lecture on Statistical Learning in Computational Biology.
- 27th of February 2016: Our paper on HLA footprints in transmitted HIV sequences got accepted at Nature Medicine.
- 2015 was a new max for program committee memberships. Nico was PC member of ISMB/ECCB 2015 (Disease models & Epidemiology track), ISMB/ECCB 2015 (Applied Bioinformatics track), MLCB 2015, GCB 2015, PSB 2016 (Precision Medicine track) , and Recomb 2016
- 19th of October 2015: The new semester started. Check out our seminar on Viral Bioinformatics.
- 28th of September 2015: Anna gave a talk on how to predict and visualize resistance to broadly neutralizing antibodies against HIV at GCB 2015.
- 27th of September 2015: Nico gave the first ever junior research group talk at GCB 2015, presenting the statistical learning in computational biology group.
- 31st of July 2015: Our manuscript (by Anna and Nico) on how to predict and visualize resistance to broadly neutralizing antibodies against HIV is accepted for presentation at GCB 2015.
- 18th of April 2015: Our manuscript (by Nora and Nico) on how to best integrate different omics data sets is accepted for presentation at ISMB/ECCB 2015.
- 25th of March 2015: The website for the SS2015 lecture 'Statistical Learning in Computational Biology' is online at https://bioinf.mpi-inf.mpg.de/teaching/slcb_2015.php.
- 14th of February 2015: Our manuscript (by Nora and Nico) on how to best integrate different omics data sets is conditionally accepted for presentation at ISMB/ECCB 2015.
- 28th of November 2014: Nico gave a talk about broadly neutralizing antibodies against HIV and associated resistance patterns at the 12th HIV/HCV Resistance Workshop, Cologne, Germany.
- 20th of November 2014: We got our recent work on broadly neutralizing antibodies against HIV accepted for presentation at CROI 2015. Anna will present it at a poster.
- 14th of October 2014: Nico gave a talk at the 3rd MoSiPS meeting - ''Biological modeling - a mirror image of reality'' Saarbrücken, Germany
- 9th of September 2014: Adrin presented our work on more robust association prediction and better visualization of important factors at the 14th Workshop on Algorithms in Bioinformatics at Wroclaw, Poland
- 6th of September 2014: Matthias presented our work on detecting DNA methylation call differences at the 3rd European Student Council Symposium in Strasbourg, France
- 2nd of June 2014: Nico gave a talk at the UniGR workshop ''Systems Biology'' at the Luxembourg Center for Systems Biomedicine
- 5th of April 2014: Nico gave two talks at the Arevir-GenaFor-Meeting 2014 at Cologne, Germany
- 26th of March 2014: Nico gave a talk at the EucoHIV Educational Workshop preceeding the 12th European Workshop on HIV & Hepatitis - Treatment Strategies & Antiviral Drug Resistance at Barcelona, Spain